More and more companies across different industries are turning to artificial intelligence (AI) to support functions. AI finds everyday use in processes such as delivering personalized customer experiences, streamlining and improving operations, increasing productivity and facilitating faster and better decisions. Projections reveal that the global AI hardware, software, and services market is expected to exceed $500 billion by 2024.
Companies often expect that once an AI system is implemented, it will stay brilliant and work magically. The need for human intervention baffles companies after investing considerable time and money in their AI systems. Another challenge lies in integrating rudimentary AI into enterprise solutions. In such instances, these AI technologies are static and remove control over data parameters that most organizations rely on. Even for the most AI-inclined organizations, there must be humans within the loop to minimize and avoid risks and maximize the benefits of AI. Let’s explore the roles and impact of human intervention in AI systems.
AI Checks and Balances
The importance of humans in the AI loop is regulatory, ethical, and reputational. Having people within the AI system will help safeguard against inaccurate data that may lead to poor decisions and other adverse outcomes. Biases with AI systems can occur during training of the AI model or as a trending bias as the AI system increasingly reacts to recent activities compared to previous actions. An AI system cannot understand the intricacies of a moral decision.
For example, AI in the healthcare industry indicates how AI and humans can work together to improve outcomes. Without human intervention, AI can cause great harm, especially in decision-making. AI recommends the doctor, who then determines if the recommended option is sound.
People must continuously monitor AI accuracy and responses to reduce the incidence of flaws that may lead to harm or catastrophe. Humans have a role to play in constantly training AI models to ensure they get better. We are already seeing a move by top companies using AI to have formal programs that monitor their digital trustworthiness.
Models for Human-AI Collaboration
Human-in-the-loop (HitL) reinforcement learning and Conversational AI is two great examples of how human intervention supports AI systems to make better decisions.
HitL reinforcement learning permits AI systems to take advantage of machine learning to learn by observing human behavior in real-life work and use cases. These systems rely on human feedback and augment human interactions for continuous self-development and improvement. HitL offers a controlled environment that reduces and eliminates the inherent risk of biases and associated consequences in the decision-making process.
The benefits of the HitL reinforcement learning model are best seen in industries that manufacture critical vehicle and aircraft parts, especially areas where equipment must meet very high standards. In these particular situations, machine learning increases the speed and accuracy of inspections while human intervention assures that manufactured parts are safe and secure for passengers.
Conversational AI offers near-human-like communications. It helps ease workloads on employees by handling more straightforward tasks and knowing when to escalate more complex issues to humans for decision making. The best example of this model is contact centers.
Customers will reach out for assistance to a contact center through the available channels, including calls, texts, or a chat with a customer care representative. The virtual agent will listen and understand the customer’s needs and engage in a back-and-forth conversation. The virtual agent will use machine learning and AI to decide how to move forward and what needs to be done based on what it learned from prior experience. Many AI systems within the contact centers can generate speech to help communicate with the customer and imitate the feeling of a human typing or talking on the other side.
In most cases, the virtual agent will sufficiently help customers resolve their problems. However, there are complex situations in which the AI system cannot continue typing or talking and will make a seamless transfer to a live representative. The live representative will take over the call or chat and resolve the customer’s problem. In such scenarios, the AI system can shift to augmentation from automation by listening to the conversation and offering recommendations that aid the live representative in making the best decision.
The combination of conversational AI and cognitive AI can help the systems understand the customer’s emotional state at the other end of the line. Other benefits include the handling of complex dialogue and interactions, providing real-time translation, and can even adjust to the behavior of the person on the line. Conversational AI with cognitive AI will take human assistance to the next level of sophistication.
The Blend of Automation and Human Interaction Leads to augmented Intelligence.
The best applications of AI are seed, where AI systems are monitored by people and augment people. In these applications, AI helps people improve their skills, move up the skill continuum, and empower them to take on more complex challenges. The AI system will continually learn and improve while constantly being kept in check to prevent risks and potentially harmful consequences.
Different models such as cognitive AI, conversational AI, and HitL reinforcement learning combined with real people with expertise, empathy, ingenuity, and moral judgment will lead to augmented Intelligence and increasingly more positive outcomes.
Author: Alessandro Civati
Blockchain ID: https://x88.life/cOD14WdXnJ
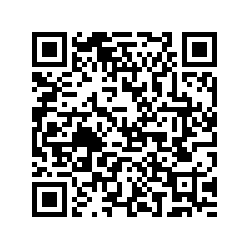